Business Case: Data Quality – Missing Process!
Introduction
First of all, let me ask you a question. What is the most vulnerable part of Data Quality process in corporation?
Some answers may be around technical aspects, like: ‘It is difficult to evaluate data’. Certainly that is true. Mostly when volume require Big Data techniques and infrastructure. There is no doubt that this is big project, require highly trained professionals and modern infrastructure.
So, it is the correct answer to question that was asked? No. Please, give me two minutes for brief introduction to Data Quality Process, then the answer will be easier to accept.
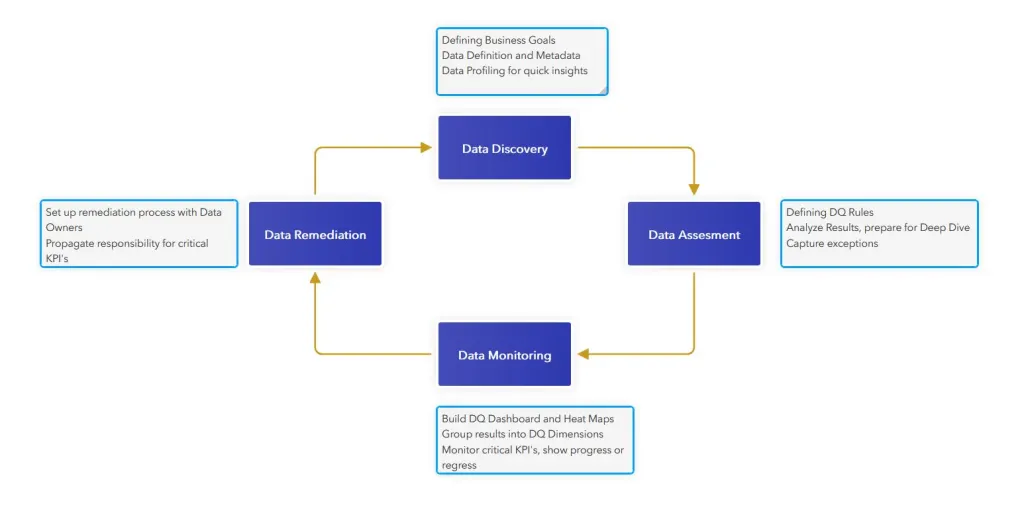
Discovery in Data Quality Process
There are multiple steps in this process. Most of them are pretty obvious when you start to think about Data Quality. Let me focus on two most important ones. First is awareness of data quality and fair discovery of consequences of poor quality of organization’s data. As my experience shows, people are aware of this consequences but on different levels. Data Stewards are focus on processing quotes and some data quality issues mostly, add precious time to process single case. Process leaders in back office hears about Data Quality when someone wrongly entered data made huge impact on insurance policy, so client or front office discovered it (before or after there is becoming financial impacts due to huge payment or missing coverage for other institutions) . As I mentioned here other institutions, there are regulations and government institutions that obligate Insurance Company for proper DQ management. Regulations are last option, but sometimes government needs to repair some problems in industry sectors. Therefore, Top Management sooner or later will be into DQ process within company.
Refining and Continous Improvement
Great, so where is the issue there? How to cope with multiple expectations and lack of big picture? How to prepare Data Quality Process Owner for this task, when DQ process requires to work from bottom to top, from Data Steward that type data into forms (or client directly is filling data but understanding of data definitions is different between company and client) to ETL processes that feed Data Warehouse for Analytical Applications? There is no simple answer for that.
Secondly, there is Data Remediation Process. I have been to many discussions where parties made simple solution to deal with any Data Quality issue with single statement. Let’s start evaluate data during entering process (client forms or operational tool currently used in company). Then, there is always similar list of problems: we have more than one system in company. This checks will kill system performance. We do not own internal code to make those changes. Cost of technical implementations will be huge (Actually, there is a cost of bad Data Quality in organization. No one knows it on those meetings), etc. I stop here.
Even when we have Data Monitoring and perfectly prepared Exception Report (that includes wrong and expected values, with clear link to spot where it should be corrected, and by whom), it is not easy task to correct data in source system.
Finally, I can reveal the answer. Organizations don’t know who is actual Data Owner, processes are so distributed, forms were filled by employees that are no longer here, or we cannot even tell what is the correct data, as no one will ask again client for policy that is closed.
If you start to think about Data Quality within your organization, let’s help you with our experience. This process will be fully covered by my team. We will join you in this discussion with Board of Directors, Top Management or even with Technicians that manages your business apps.
PS. There is no better dashboard for monitoring DQ KPI’s than DQ Heat Map. This is a single tool for presenting DQ insights. This is quick check of critical company data elements. Check out this example:
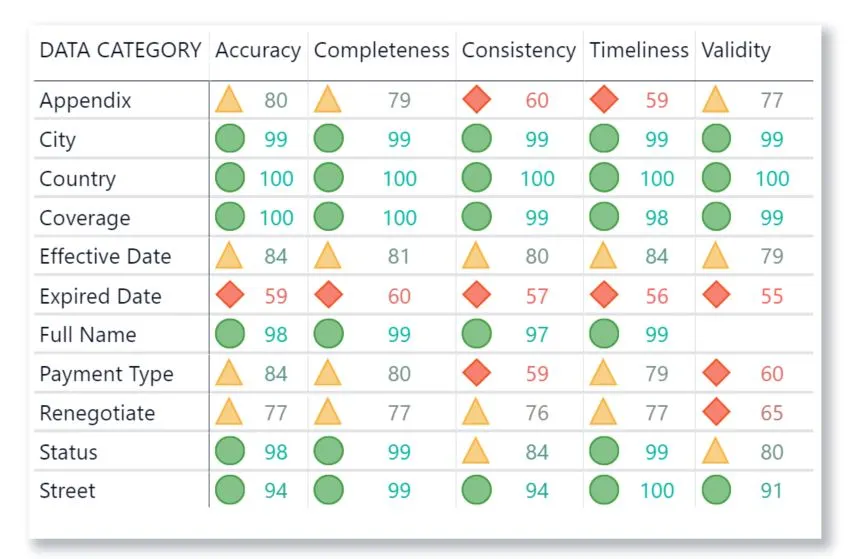
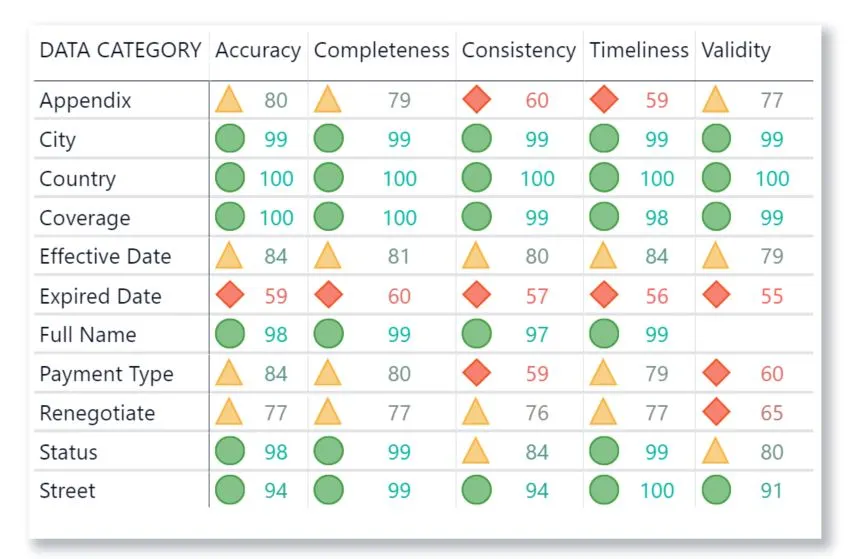
Author
Grzegorz Gruszka
Many companies see 'constant change' as baseline motto for process optimization, but people like me are those who translate that motto into action points. There is huge values in data, but my goal is to see data as an organizm, because understanding each small part does not replace big picture of organizm linkage to ecosystem. Data are part of our World. Let us start change it based on deep discoveries in data.